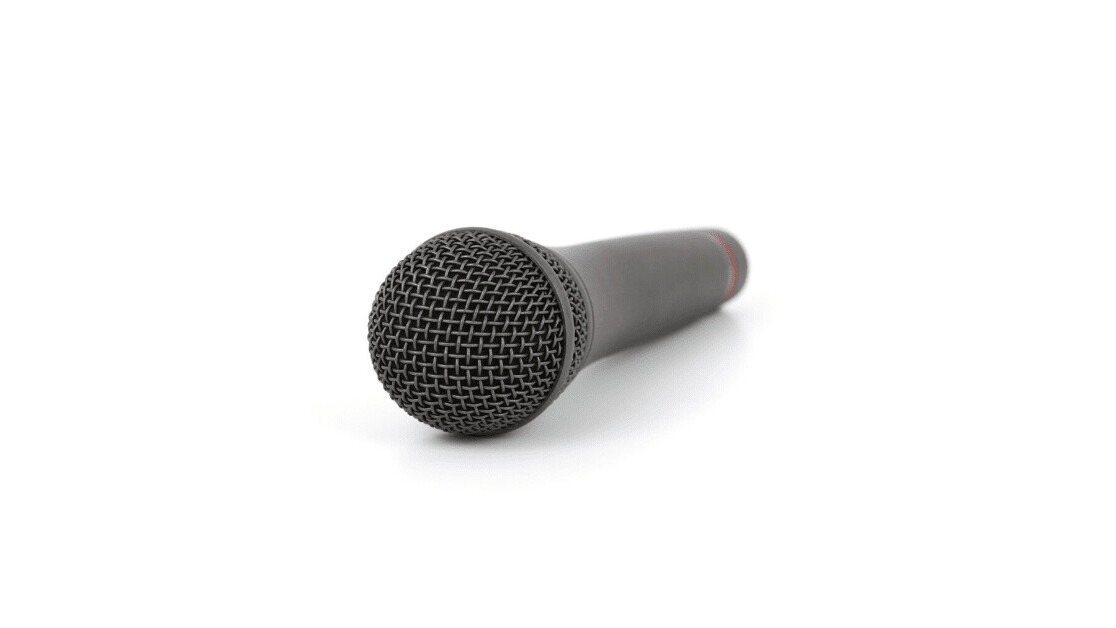
If you have ever had to repeat “yes” six times to your automated telephone banking assistant, you’d be forgiven for thinking that automatic speech recognition (ASR) has a long way yet to go. But it might surprise you to know that computers are now as good as humans at recognizing words in telephone calls.
ASR has important implications for the efficiency of businesses of all sizes — not least in complying with Subject Access Requests and helping locate business conversations. With the introduction of the General Data Protection Regulation in 2018, individuals are increasingly aware of their rights. One of these is the right of customers, suppliers and employees to request to see what data a company holds about them.
But before we discuss more about ASR, let me clarify the difference between speech and voice.
[Read: 2020 will mark the death of the chatbot as we know it]
The term “voice assistant” has been coined to describe devices and services for acting on the spoken word. It’s not a big jump to wrongly describe what they do as voice recognition. However, it is speech they are attempting to recognize, not voice. Voice recognition is about discovering who is speaking. Speech recognition is about what they are saying.
Context is king
In my early career, undertaking ASR research for a well-funded government laboratory, computers had less than 1,000th the power of an iPhone. Number crunching solutions were therefore not an option. Instead, we tried to work out what the human was doing and see if we could learn from that. We discovered that much of the information we, as humans, use to understand speech is not just in the acoustic sound but in its context.
For example, when we speak English, most of us follow the rules of English grammar. So that although we may find it difficult to discriminate between, say, “nine” and “mine,” we use context, including the rules of grammar, to help us. Hearing “I would like mine bags,” doesn’t make sense. It would in most circumstances be incorrect to say “mine bags,” so either they said “my” or “nine.”
In a stationery shop, “nine” makes sense, if at airport customs, “my” makes more. Whatever we hear, we subconsciously change the “mine” to “my” or “nine” depending on the situation. But with random words (no grammar, no context), humans are just as likely to get them wrong as the computer.
Grammar and context are just two tools we can use to improve performance. There are others including linguistics, semantics, and phonetics. Indeed, there are many other disciplines that could help, but to apply these, a programmer would need to understand what they are and how they affect the understanding of speech.
Herein lies the rub. We don’t teach grammar to children to get them to speak. At five they are saying:
“I cutted the paper”.
Without a single grammar lesson, by six they are saying,
“I cut the paper”
Children learn by hearing lots of adults speak and eventually, their brain refines the grammar rules and remembers the exceptions.
The temptation has been to let the computer do the same. One way is by using techniques like neural networks which, loosely speaking, attempt to simulate the operation of the human brain — using significant computing resources. Providers don’t want every “voice assistant” to have to learn from scratch, so they build-in rules pre-learnt from listening to millions of hours of human speech.
Because the neural networks “unconsciously” learn rules about phonetics, linguistics, etc., programmers do not need to know how those rules are interpreted — just like the five year old.
The business drive is therefore to get computers to self-learn the rules, which is now viable because computing power is so plentiful and cheap — unlike speech experts. But if the device fails to recognize speech correctly, there is no easy way to fix it other than get it to listen to lots more samples.
Unfortunately, just as they fail to publish performance statistics, the providers of ASR services fail also to publish their ASR methods. Applying my experience in ASR research when examining some of the best ASR systems leads me to believe that over-relying on neural network approaches is common.
Whatever the reason, while our devices may be good at recognizing words, they are not yet so good at actually understanding speech.
As it is beyond most company’s resources to develop ASR systems from scratch, can we improve them with contextual information we already have?
That vital contextual information is not available to the ASR service providers, nor could they reasonably be expected to apply it for such a general requirement. One such source specific to each business is email correspondence. It will be no surprise that we say things that we write, so our emails are stacked with context. And once our speech is correctly understood, that in turn, provides the vital context for other business knowledge.
The technology to achieve this is already here and 2020 should see this take off. And by take off, I mean get high traction in small businesses.
The practicalities
To use ASR on telephone calls, we must first capture the call in a digital form. The choice then is between processing it in-house or via a Cloud service. The trend is towards Cloud services because they are able to share extremely large (and costly) processing power among many users. Without an internet connection, Siri and Alexa would be useless.
Five years ago, neither of these options were viable for SMEs. But now with VoIP telephony and fast internet, it is possible to ingest phone calls and dispatch them to a Cloud-based ASR service for processing in real time. With transcription costs of £3ish per hour (and dropping), it is feasible for a small company to routinely transcribe all calls.
However, except for ad-hoc requirements, it is not feasible for employees to manually locate calls that they need transcribing then transfer them to the Cloud service provider for processing. To do so, the employee must know how to capture, find, format, and upload the call, then download and file the result, not to mention have an account with the service provider.
But this is all set to change with sophisticated technology and services that can automatically aggregate several information sources that can be easily searched just minutes after calls are completed. And by obtaining context from other types of message such as emails, we hope to see real speech understanding.
Some ASR providers are starting to see the value of context, but for a generation of developers oblivious to the underlying value of a theoretical basis for speech understanding, things will have to change.
Capture if you can
So what does this mean for businesses today? As the technology quickly evolves, we should capture every call we can. Any company, no matter how small, cannot afford not to do this. Speech understanding by computer will eventually catch up with and even surpass the performance of a human. If we wait until then before storing our phone calls, then we will lose the ability to search and retrieve historically important calls and to capitalize on technologies like SEO voice search.
More importantly, we will lose with it the vital intelligence in those calls. It is time for all businesses to think about the future of voice technology, and what it could mean for them. Wait until ASR is perfect and you may miss the boat.
Get the TNW newsletter
Get the most important tech news in your inbox each week.