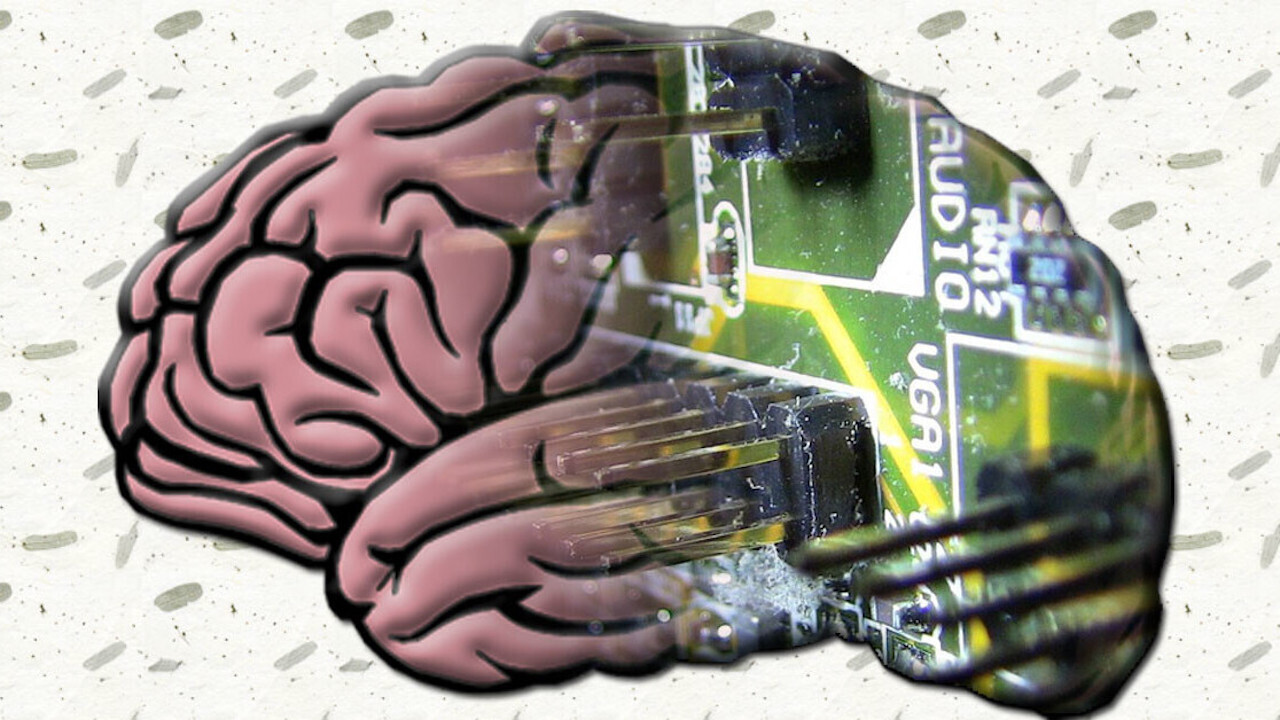
The past month has seen a frenzy of articles, interviews, and other types of media coverage about Blake Lemoine, a Google engineer who told The Washington Post that LaMDA, a large language model created for conversations with users, is “sentient.”
After reading a dozen different takes on the topic, I have to say that the media has become (a bit) disillusioned with the hype surrounding current AI technology. A lot of the articles discussed why deep neural networks are not “sentient” or “conscious.” This is an improvement in comparison to a few years ago, when news outlets were creating sensational stories about AI systems inventing their own language, taking over every job, and accelerating toward artificial general intelligence.
But the fact that we’re discussing sentience and consciousness again underlines an important point: We are at a point where our AI systems—namely large language models—are becoming increasingly convincing while still suffering from fundamental flaws that have been pointed out by scientists on different occasions. And I know that “AI fooling humans” has been discussed since the ELIZA chatbot in the 1960s, but today’s LLMs are really at another level. If you don’t know how language models work, Blake Lemoine’s conversations with LaMDA seem almost look surreal—even if they had been cherry-picked and edited.
However, the point I want to make here is that “sentience” and “consciousness” is not the best discussion to have about LLMs and current AI technology. A more important discussion would be one about human compatibility and trust, especially since these technologies are being prepared to be integrated into everyday applications.
Why large language models don’t speak our language
The workings of neural networks and large language models have been thoroughly discussed in the past week (I strongly recommend reading Melanie Mitchell’s interview with MSNBC for a balanced account of how LaMDA and other LLMs work). I would like to give a more zoomed-out view of the situation, starting with human language, with which LLMs are compared.
For humans, language is a means to communicate the complicated and multi-dimensional activations happening in our brains. For example, when two brothers are talking to each other and one of them says “mom,” the word is associated with a lot of activations in different parts of the brain, including memories of her voice, face, feelings, and different experiences from the distant past to (possibly) recent days. In fact, there might be a huge difference between the kind of representations that the brothers hold in their brains, depending on the experiences that each has had. The word “mom,” however, provides a compressed and well-represented approximation that helps them agree on the same concept.
When you use the word “mom” in a conversation with a stranger, the difference between the experiences and memories becomes even wider. But again, you manage to reach an agreement based on the shared concepts that you have in your minds.
Think of language as a compression algorithm that helps transfer the enormous information in the brain to another person. The evolution of language is tied directly to experiences we’ve had in the world, from physical interactions in our environment to social interactions with other fellow humans.
Language is built on top of our shared experiences in the world. Children know about gravity, dimension, physical consistency of objects, and human and social concepts such as pain, sadness, fear, family, and friendship even before uttering their first word. Without those experiences, language has no meaning. This is why language usually omits commonsense knowledge and information that interlocutors share. On the other hand, the level of shared experience and memory will determine the depth of conversation you can have with another person.
In contrast, large language models have no physical and social experience. They are trained on billions of words and learn to respond to prompts by predicting the next sequence of words. This is an approach that has yielded great results in the past few years, especially after the introduction of the transformer architecture.
How do transformers manage to make very convincing predictions? They turn text into “tokens” and “embeddings,” mathematical representations of words in a multi-dimensional space. They then process the embedding to add other dimensions such as the relations between the words in a sequence of text and their role in the sentence and paragraph. With enough examples, these embeddings can create good approximations of how words should appear in sequences. Transformers have become especially popular because they are scalable: Their accuracy improves as they become larger and are fed on more data, and they can be mostly trained through unsupervised learning.
But the fundamental difference remains. Neural networks process language by turning them into embeddings. For humans, language is the embedding of thoughts, feelings, memory, physical experience, and many other things that we have yet to discover about the brain.
This is why it is fair to say that despite their immense advances and impressive results, transformers, large language models, deep neural networks, etc. are still far from speaking our language.
Sentience vs compatibility and trust
A lot of the discussions today are about whether we should assign attributes such as sentience, consciousness, and personhood to AI. The problem with these discussions is that they are focused on concepts that are vaguely defined and mean different things to different people.
For example, functionalists might argue that neural networks and large language models are conscious because they manifest (at least in part) the same kind of behavior that you would expect from a human, even though they are built on a different substrate. Others might argue that organic substance is a requirement for consciousness and conclude that neural networks will never be conscious. You can throw in arguments about qualia, the Chinese room experiment, the Turing test, etc., and the discussion can go on forever.
However, a more practical question is, how “compatible” are current neural networks with the human mind, and how far can we trust them with critical applications? And this is an important discussion to have because large language models are mostly developed by companies that seek to turn them into commercial applications.
For example, with enough training, you might be able to train a chimpanzee to ride a car. But would you put it behind a steering wheel on a road that pedestrians will be crossing? You wouldn’t, because you know that however smart they are, chimpanzees don’t think in the same way as humans and can’t be given responsibility for tasks where human safety is concerned.
Likewise, a parrot can be taught many phrases. But would you trust it to be your customer service agent? Probably not.
Even when it comes to humans, some cognitive impairments disqualify people from taking on certain jobs and tasks that require human interactions or regard human safety. In many cases, these people can read, write, speak fluently, and remain consistent and logical in lengthy conversations. We don’t question their sentience or consciousness or personhood. But we know that their decisions can become inconsistent and unpredictable due to their illness (see the case of Phineas Gage, for example).
What matters is whether you can trust the person to think and decide as an average human would. In many cases, we trust people with tasks because we know that their sensory system, common-sense knowledge, feelings, goals, and rewards are mostly compatible with ours, even if they don’t speak our language.
What do we know about LaMDA? Well, for one thing, it doesn’t sense the world as we do. Its “knowledge” of language isn’t built on the same kind of experiences as ours. Its commonsense knowledge is built on an unstable foundation because there’s no guarantee that large amounts of text will cover all the things we omit in language.
Given this incompatibility, how far can you trust LaMDA and other large language models, no matter how good they are at producing text output? A friendly and entertaining chatbot program might not be a bad idea as long as it doesn’t steer the conversation into sensitive topics. Search engines are also a good application area for LLMs (Google has been using BERT in search for a few years). But can you trust them with more sensitive tasks, such as an open-ended customer service chatbot or a banking advisor (even if they have been trained or finetuned on a ton of relevant conversation transcripts)?
My thinking is that we’ll need application-specific benchmarks to test the consistency of LLMs and their compatibility with human common sense in different areas. When it comes to real applications, there should always be clearly defined boundaries that determine where the conversation becomes off-limits for the LLM and should be handed to a human operator.
The problem-solver perspective
A while back, I wrote an essay on “problem finders” and “problem solvers.” Basically, what I said is that human intelligence is about finding the right problems and artificial intelligence (or the AI we have today) is about solving those problems in the most efficient manner.
We have seen time and again that computers are able to find shortcuts for solving complicated problems without acquiring the cognitive abilities of humans. We’ve seen it with checkers, chess, Go, programming contests, protein folding, and other well-defined problems.
Natural language is in some ways different but also similar to all those other problems AI has solved. On the one hand, transformers and LLMs have shown that they can produce impressive results without going through the process of learning language like a normal human, which is to first explore the world and understand its basic rules and then acquire the language to interact with other people based on this common knowledge. On the other hand, they lack the human experience that comes with learning language. They can be useful for solving well-defined language-related problems. But we should not forget that their compatibility with human language processing is limited and thus we should be careful how far we trust them.
This article was originally published by Ben Dickson on TechTalks, a publication that examines trends in technology, how they affect the way we live and do business, and the problems they solve. But we also discuss the evil side of technology, the darker implications of new tech, and what we need to look out for. You can read the original article here.
Get the TNW newsletter
Get the most important tech news in your inbox each week.