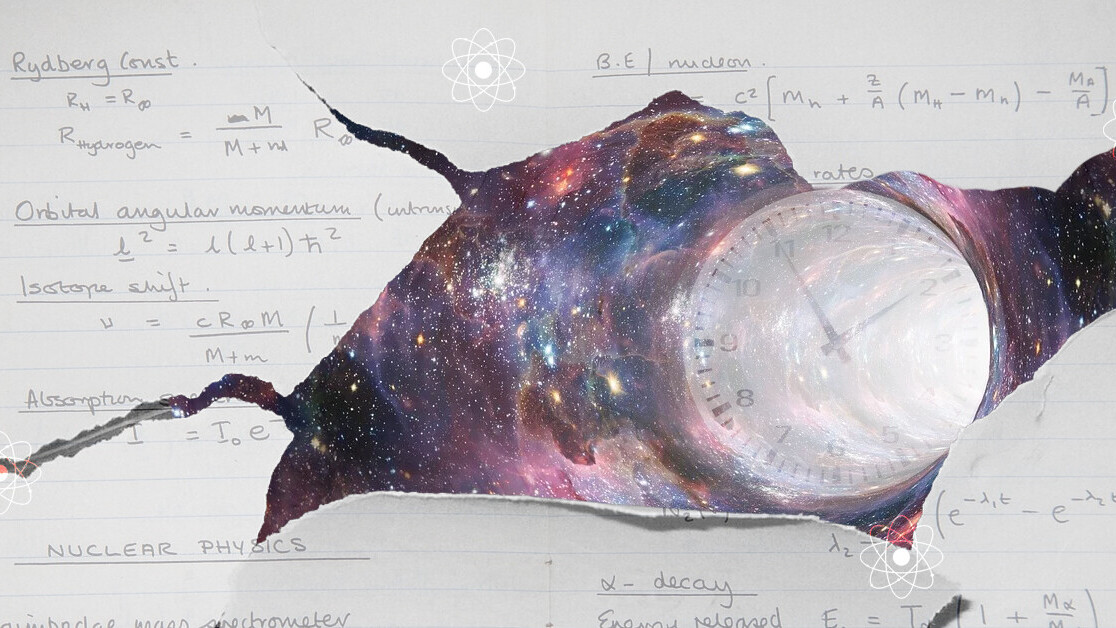
We’ve painted ourselves into another corner with artificial intelligence. We’re finally starting to breakthrough the usefulness barrier but we’re butting up against the limits of our our ability to responsibly meet our machines’ massive energy requirements.
At the current rate of growth, it appears we’ll have to turn Earth into Coruscant if we want to keep spending unfathomable amounts of energy training systems such as GPT-3 .
The problem: Simply put, AI takes too much time and energy to train. A layperson might imagine a bunch of code on a laptop screen when they think about AI development, but the truth is that many of the systems we use today were trained on massive GPU networks, supercomputers, or both. We’re talking incredible amounts of power. And, worse, it takes a long time to train AI.
The reason AI is so good at the things it’s good at, such as image recognition or natural language processing, is because it basically just does the same thing over and over again, making tiny changes each time, until it gets things right. But we’re not talking about running a few simulations. It can take hundreds or even thousands of hours to train up a robust AI system.
One expert estimated that GPT-3, a natural language processing system created by OpenAI, would cost about $4.6 million to train. But that assumes one-shot training. And very, very few powerful AI systems are trained in one fell swoop. Realistically, the total expenses involved in getting GPT-3 to spit out impressively coherent gibberish are probably in the hundreds-of-millions.
GPT-3 is among the high-end abusers, but there are countless AI systems out there sucking up hugely disproportionate amounts of energy when compared to standard computation models.
The problem? If AI is the future, under the current power-sucking paradigm, the future won’t be green. And that may mean we simply won’t have a future.
The solution: Quantum computing.
An international team of researchers, including scientists from the University of Vienna, MIT, Austria, and New York, recently published research demonstrating “quantum speed-up” in a hybrid artificial intelligence system.
In other words: they managed to exploit quantum mechanics in order to allow AI to find more than one solution at the same time. This, of course, speeds up the training process.
Per the team’s paper:
The crucial question for practical applications is how fast agents learn. Although various studies have made use of quantum mechanics to speed up the agent’s decision-making process, a reduction in learning time has not yet been demonstrated.
Here we present a reinforcement learning experiment in which the learning process of an agent is sped up by using a quantum communication channel with the environment. We further show that combining this scenario with classical communication enables the evaluation of this improvement and allows optimal control of the learning progress.
How?
This is the cool part. They ran 10,000 models through 165 experiments to determine how they functioned using classical AI and how they functioned when augmented with special quantum chips.
And by special, that is to say, you know how classical CPUs process via manipulation of electricity? The quantum chips the team used were nanophotonic, meaning they use light instead of electricity.
The gist of the operation is that in circumstance where classical AI bogs down solving very difficult problems (think: supercomputer problems), they found the hybrid-quantum system outperformed standard models.
Interestingly, when presented with less difficult challenges, the researchers didn’t not observe any performance boost. Seems like you need to get it into fifth gear before you kick in the quantum turbocharger.
There’s still a lot to be done before we can roll out the old “mission accomplished” banner. The team’s work wasn’t the solution we’re eventually aiming for, but more of a small-scale model of how it could work once we figure out how to apply their techniques to larger, real problems.
You can read the whole paper here on Nature.
H/t: Shelly Fan, Singularity Hub
Get the TNW newsletter
Get the most important tech news in your inbox each week.