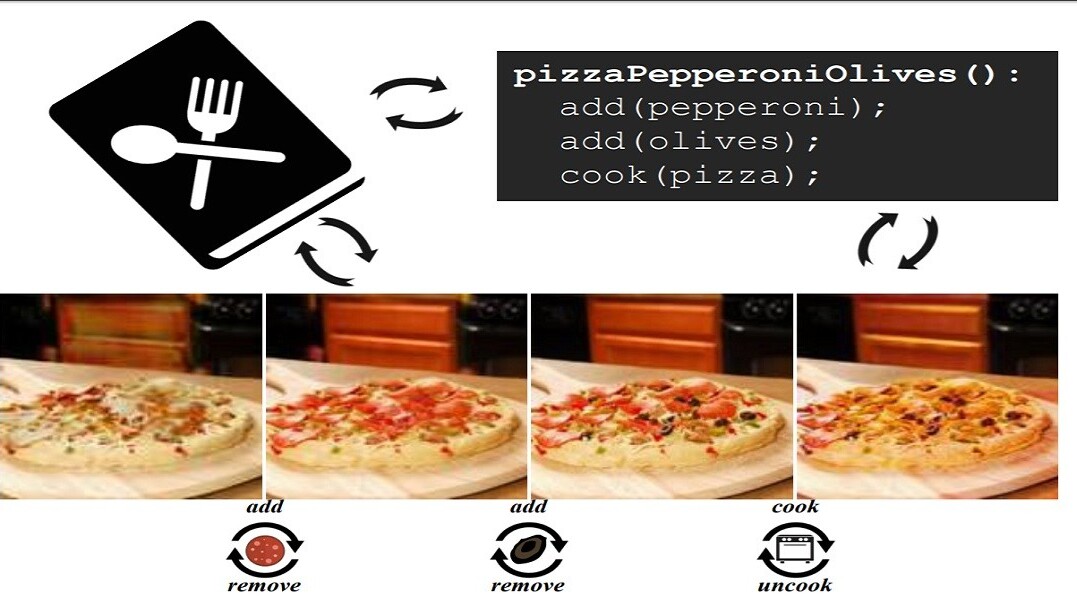
A group of MIT researchers recently developed an AI model that takes a list of instructions and generates a finished product. The future implications for the fields of construction and domestic robotics are huge, but the team decided to start with something we all need right now: pizza.
PizzaGAN, the newest neural network from the geniuses at MIT’s Computer Science and Artificial Intelligence Laboratory (CSAIL) and the Qatar Computing Research Institute (QCRI), is a generative adversarial network that creates images of pizza both before and after it’s been cooked.
No, it doesn’t actually make a pizza that you can eat – at least, not yet. When we hear about robots replacing humans in the food industry we might imagine a Boston Dynamics machine walking around a kitchen flipping burgers, making fries, and yelling “order up,” but the truth is far more tame.
In reality these restaurants use automation, not artificial intelligence. The burger-flipping robot doesn’t care if there’s an actual burger or a hockey puck on its spatula. It doesn’t understand burgers or know what the finished product should actually look like. These machines would be just at home taping boxes shut in an Amazon warehouse as they are at a burger joint. They’re not smart.
What MIT and QCRI have done is create a neural network that can look at an image of a pizza, determine the type and distribution of ingredients, and figure out the correct order to layer the pizza before cooking. It understands – as much as any AI understands anything – what making a pizza should look like from start to finish.
The joint team accomplished this through a novel modular approach. It developed the AI with the ability to visualize what a pizza should look like based on whether ingredients have been added or removed. You can show it an image of a pizza with the works, for example, and then ask it to remove mushrooms and onions and it’ll generate an image of the modified pie.
According to the researchers:
From a visual perspective, every instruction step can be seen as a way to change the visual appearance of the dish by adding extra objects (e.g., adding an ingredient) or changing the appearance of the existing ones (e.g., cooking the dish).
In order for a robot or machine to one day make a pizza in the real world, it’ll have to understand what a pizza is. And so far humans, even the really smart ones at CSAIL and QCRI, are way better at replicating vision in robots than taste buds.
Domino’s pizza, for example, is currently testing a computer vision solution to quality control. It’s using AI in some locations to monitor every pizza coming out of the ovens to determine if they look good enough to meet the company’s standard. Things like topping distribution, even cooking, and roundness can be measured and quantified by machine learning in real-time to ensure customers don’t get a crappy pie.
MIT and QCRI’s solution integrates the pre-cooking phase and determines the proper layering to make a tasty, appealing pizza. At least in theory – we could be years away from an end-to-end AI-powered solution for preparing, cooking, and serving pizza.
Of course, pizza isn’t the only thing that a robot could make once it understands the nuances of ingredients, instructions, and how the end-result of a project should appear. The researchers concluded the underlying AI models behind PizzaGAN could be useful in other domains:
Though we have evaluated our model only in the context of pizza, we believe that a similar approach is promising for other types of foods that are naturally layered such as burgers, sandwiches, and salads. Beyond food, it will be interesting to see how our model performs on domains such as digital fashion shopping assistants, where a key operation is the virtual combination of different layers of clothes.
But, let’s be honest, we won’t officially enter the AI era until the day arrives that we can get a decent brick-oven Margherita pizza made to-order by a self-contained robot.
H/t: George Sief, Towards Data Science.
Get the TNW newsletter
Get the most important tech news in your inbox each week.