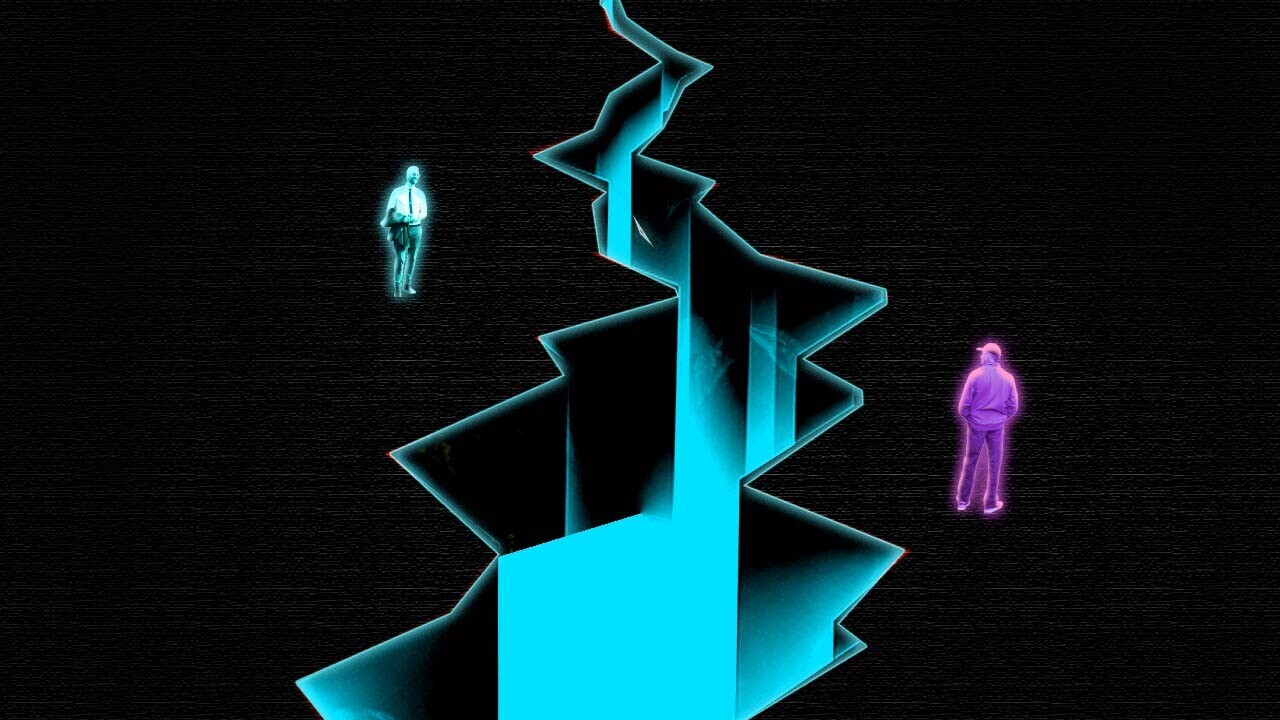
No one in the C-suite cares which coding language is chosen by engineers and data scientists — until the decision affects the bottom line.
While many have made a case about why low-code is well-suited for the data scientist, I think there may be an equally strong case to be made for the benefits of the business.
Data science is a collaborative art — one that requires a combination of data and business acumen. Yet, in reality, the two departments couldn’t sit farther apart. While data scientists worry about feature selection and model accuracy, their business counterparts think about customer retention and product quality. While data scientists are concerned with classifications, business folks are concerned about balance sheets.
Choosing low-code software for data science is investing in a common ground between the data scientists and the business unit — who are the “end-users.” It’s investing in more efficient working groups and in knowledge sharing and upskilling. Most importantly, it’s investing in getting data to quickly power many more decisions in your organization.
The importance of Time-to-Eureka!
Every data scientist is driven by the ‘Eureka!’ moment. It’s the instant when they’ve made sense of data and can leverage that newfound sense into something bigger, like predicting future buying habits. That eureka moment is also when others in the organization start to see and understand the value of data science.
The more time it takes for anyone in the organization to understand the value of data (Time-to-Eureka!), the harder it is for the data science team to work. They waste time explaining, documenting, and advocating for their work, while projects get delayed, blocked, or canceled.
On the flip side, business users are not exposed to enough problems to know what questions to ask or whether this function has value at all.
In other words, a short Time-to-Eureka! is the linchpin to scaling the function of data science in the modern enterprise. When adopted across the enterprise, a low-code tool has two positive effects.
First, more people in the organization understand what can be done with data and, therefore, know better what questions can be asked of it. Second, more people in the organization are empowered to perform basic data science tasks themselves.
With a low-code tool, we’re no longer “just” talking about a tool that’s efficient for data scientists to do their jobs. We’re now talking about a tool that advances basic data understanding in the enterprise and makes transparent the use of the most complex technologies — including notoriously nebulous machine learning capabilities.
The cascading effect of understanding
To fully demonstrate the effects of understanding data science, it’s helpful to think about it spread along two axes:
Horizontally: Teams outside of the data science group “get” the work that the data science teams do and how they prioritize projects. This includes sales and marketing groups, finance groups, operations teams, etc.
These teams are often the ones actually closest to the data that the company gathers and thus well-positioned to ask questions of it. The more they work with efficient data science teams, the more bespoke their questions get.
Vertically: Similarly, data will start to be understood by people at various levels. Not just the data team, but the team lead, the manager, the VP, the CxO, all the way up to the CEO and the board of directors.
Since these people sit far away from the data entry points, they need to find a way to stay connected with what’s happening in the data trenches. Knowing which insights to filter up to make decisions surrounding innovation, risk mitigation, and cost savings can quickly become competitively differentiating.
The cascading effect of doing
Getting more people in the organization to “do” data science, of course, also has an effect on the organization. By upskilling makers, an organization all of a sudden 100x’d (or more) its data science bandwidth.
A Ph.D. or folks with 10+ years of experience in data mining are not the only ones who can derive insights from data. Some might call this data literacy — or creating “citizen data scientists.”
This subject necessitates an important clarification. Everyone in your organization isn’t going to become a data scientist — data science is complex stuff. Instead, low-code creates wider access to custom data science.
If you think of data science in levels, according to complexity, you might rank it as such:
- Level 4: Artificial intelligence and machine learning
- Level 3: Predictive analytics
- Level 2: Visualizations and data exploration
- Level 1: Data wrangling
Today’s data scientists are often spending an exorbitant amount of time on levels 1 and 2. When more people in the organization are able to understand how to do the lower levels of analytics, data scientists are freed up to push the team into more cutting-edge methods. The access to methodologies is lowered at the bottom and pushed at the top, for laymen and experts.
Of course, a pervasive understanding of how the data science platform works won’t really lead to a clean cut of who does what data science work. In many cases, data scientists will still help with basic problems.
However, when the end-user starts on level 1, rather than level 0, they’re able to participate in the process. They can give feedback and, in some cases, reuse and adapt past workflows to future problems.
Low-code unblocks data understanding
The use cases for data science far exceed the bandwidth of any enterprise data science team, yet even the simplest automation and ETL projects take months to realize. While it’s tempting to blame change management and corporate red tape, the true blocker to data science success is a lack of data understanding.
Low-code is not just well-suited for data science programming, it’s well-suited for bringing the business and the data science team closer together.
Get the TNW newsletter
Get the most important tech news in your inbox each week.