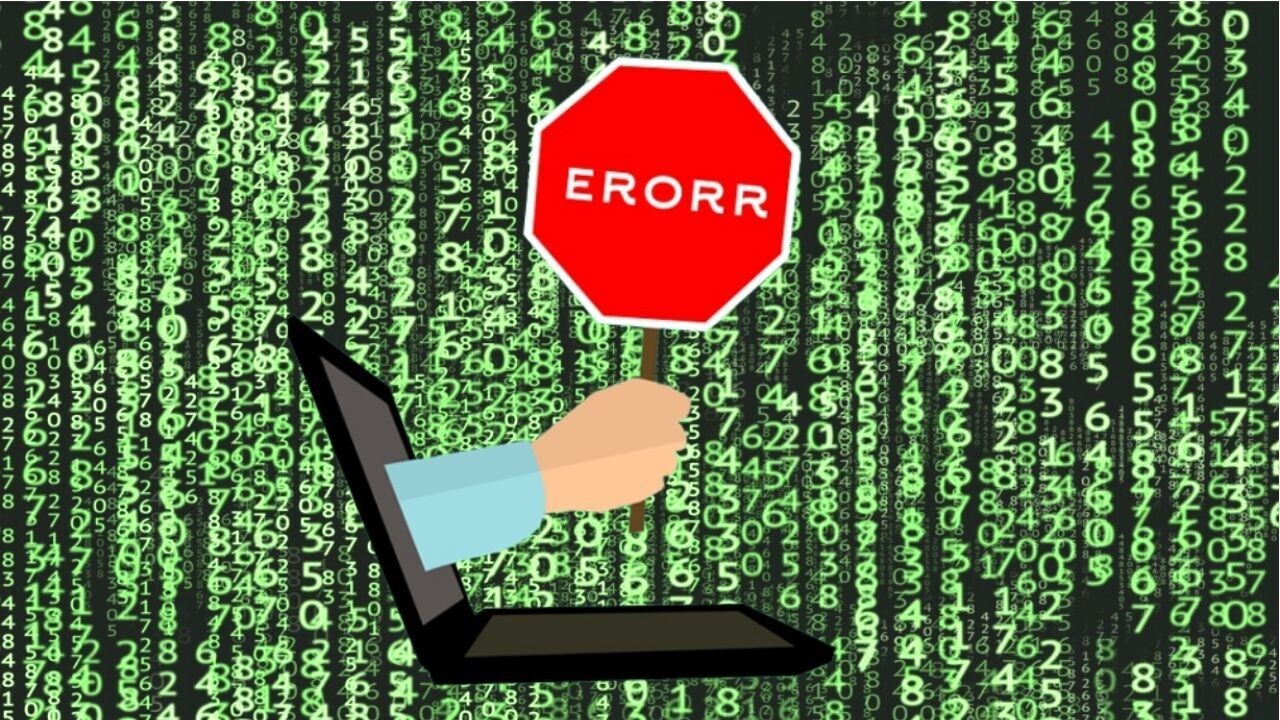
AI now guides numerous life-changing decisions, from assessing loan applications to determining prison sentences.
Proponents of the approach argue that it can eliminate human prejudices, but critics warn that algorithms can amplify our biases — without even revealing how they reached the decision.
This can result in AI systems leading to Black people being wrongfully arrested, or child services unfairly targeting poor families. The victims are frequently from groups that are already marginalized.
Alejandro Saucedo, Chief Scientist at The Institute for Ethical AI and Engineering Director at ML startup Seldon, warns organizations to think carefully before deploying algorithms. He told TNW his tips on mitigating the risks.
Explainability
Machine learning systems need to provide transparency. This can be a challenge when using powerful AI models, whose inputs, operations, and outcomes aren’t obvious to humans.
Explainability has been touted as a solution for years, but effective approaches remain elusive.
“The machine learning explainability tools can themselves be biased,” says Saucedo. “If you’re not using the relevant tool or if you’re using a specific tool in a way that’s incorrect or not-fit for purpose, you are getting incorrect explanations. It’s the usual software paradigm of garbage in, garbage out.”
While there’s no silver bullet, human oversight and monitoring can reduce the risks.
Saucedo recommends identifying the processes and touchpoints that require a human-in-the-loop. This involves interrogating the underlying data, the model that is used, and any biases that emerge during deployment.
The aim is to identify the touchpoints that require human oversight at each stage of the machine learning lifecycle.
Ideally, this will ensure that the chosen system is fit-for-purpose and relevant to the use case.
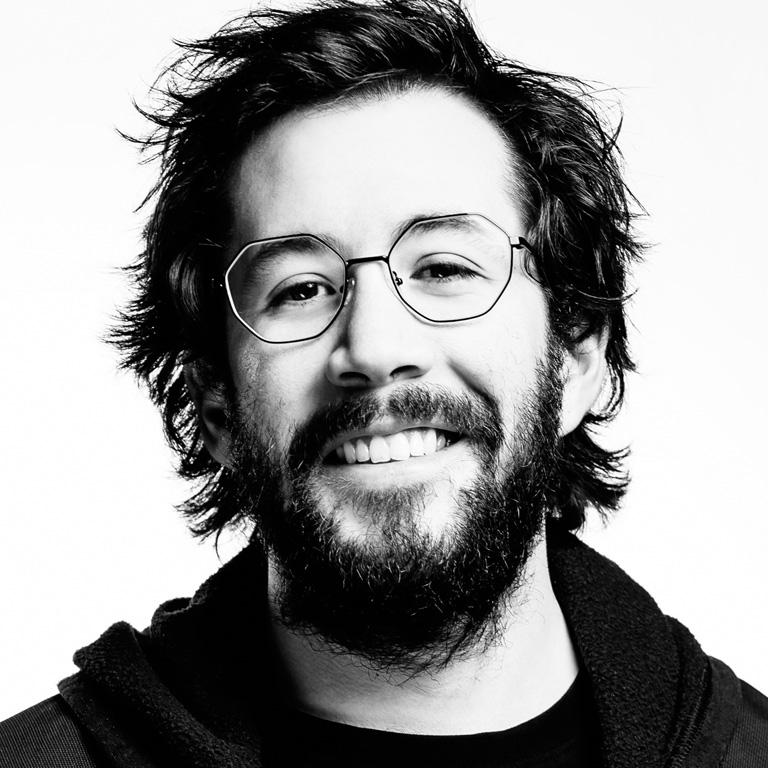
Domain experts can also use machine learning explainers to assess the prediction of the model, but it’s imperative that they first evaluate the appropriateness of the system.
“When I say domain experts, I don’t always mean technical data scientists,” says Saucedo. “They can be industry experts, policy experts, or other individuals with expertise in the challenge that’s being tackled.”
Accountability
The level of human intervention should be proportionate to the risks. An algorithm that recommends songs, for instance, won’t require as much oversight as one that dictates bail conditions.
In many cases, an advanced system will only increase the risks. Deep learning models, for example, can add a layer of complexity that causes more problems than it solves.
“If you cannot understand the ambiguities of a tool you’re introducing, but you do understand that the risks have high stakes, that’s telling you that it’s a risk that should not be taken,” says Saucedo.
The operators of AI systems must also justify the organizational process around the models they introduce.
This requires an assessment of the entire chain of events that leads to a decision, from procuring data to the final output.
You need a framework of accountability
“There is a need to ensure accountability at each step,” says Saucedo. “It’s important to make sure that there are best practices on not just the explainability stage, but also on what happens when something goes wrong.”
This includes providing a means to analyze the pathway to the outcome, data on which domain experts were involved, and information on the sign-off process.
“You need a framework of accountability through robust infrastructure and a robust process that involves domain experts relevant to the risk involved at every stage of the lifecycle.”
Security
When AI systems go wrong, the company that deployed them can also suffer the consequences.
This can be particularly damaging when using sensitive data, which bad actors can steal or manipulate.
“If artifacts are exploited they can be injected with malicious code,” says Saucedo. “That means that when they are running in production, they can extract secrets or share environment variables.”
The software supply chain adds further dangers.
Organizations that use common data science tools such as TensorFlow and PyTorch introduce extra dependencies, which can heighten the risks.
An upgrade could cause a machine learning system to break, and attackers can inject malware at the supply chain level.
The consequences can exacerbate existing biases and cause catastrophic failures.
Saucedo again recommends applying best practices and human intervention to mitigate the risks.
An AI system may promise better results than humans, but without their oversight, the results can be disastrous.
Did you know Alejandro Saucedo, Engineering Director at Seldon and Chief Scientist at the Institute for Ethical AI & Machine Learning, is speaking at the TNW Conference on June 16? Check out the full list of speakers here.
Get the TNW newsletter
Get the most important tech news in your inbox each week.