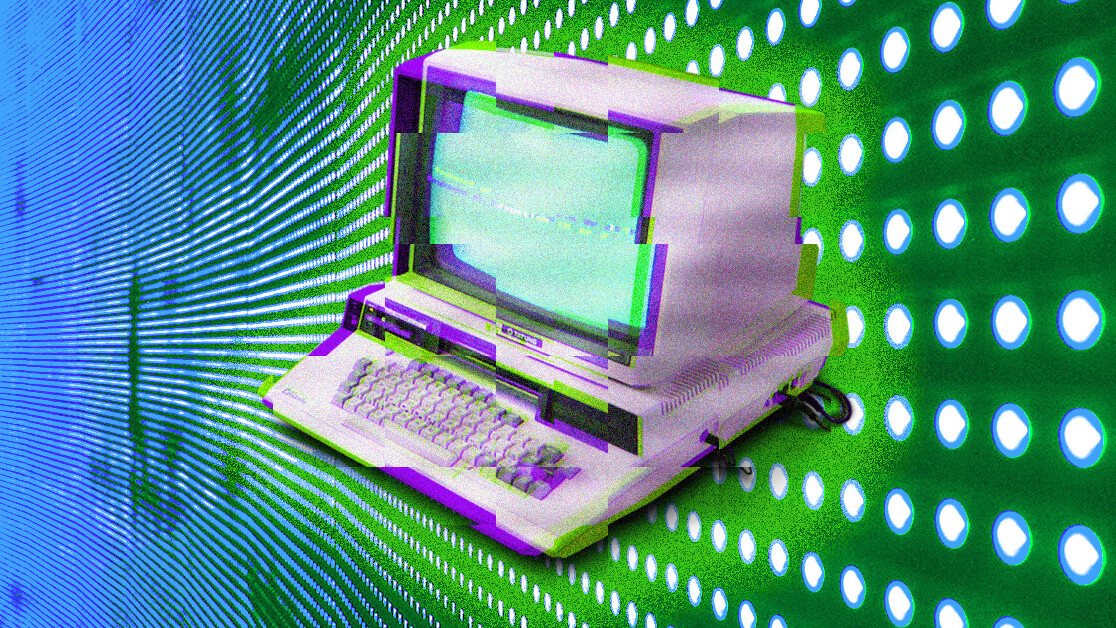
Open source software is an increasingly important part of many organizations. Yet establishing sustainable business models to support open source development is a non-trivial problem because the underlying technologies are given away for free.
But it’s still possible to build a successful business around open source software — I know, because my team already did it. KNIME has managed to establish a new business model for providing production-ready open source enterprise software, let me explain how.
Unlike business models based on selling a proprietary version of an open source software application, we went for offering two separate but complementary pieces of software.
I’ve found this allows for a clear division between the open source application and the commercial offering so that individuals have the typical open source innovation climate, while the commercial software helps the organization productionize their results in a scalable and risk-mitigated way.
[Read: The new Chinese digital consumers your business needs to target]
This approach allows individuals and organizations to stay at the forefront of an innovative field (here: data science) while at the same time productionizing what has shown to work.
It also provides a solid revenue model for the software provider. I’m happy to say KNIME, which employs more than 120 and growing, has been profitable since day one. Hopefully my experience will help you to do the same.
Open source business models
There are many ways to establish a business model around an open source software system. In real life, businesses typically use a combination of the following commercial strategies, which can be grouped into a few main categories:
- Professional services, ranging from installation and maintenance support to consulting projects involving customized solutions
- Commercial extensions, such as installers for prepackaged distributions or “open core” models that add additional, proprietary features to an open source core
- Advertising, or selling merchandise
- Hosting, generally as a cloud-based service
- Fundraising, which might include contributions from individuals or corporate sponsorships
There are other variants of more or less open source models; the corresponding Wikipedia article provides a comprehensive overview. What all models have in common, albeit to different degrees, is that they leverage the open source community for early adoption, essentially crowdsourcing many of the marketing and presales activities.
Complementing open source with commercial software
Some companies try to commercialize parts of the open source software but then have to walk a thin line between making sure they have a product that is useful even without a license for the professional version, while still providing incentives to organizations to pay for said professional license.
This is more easily accepted by users if the commercial add-on pieces are only useful in a corporate or enterprise environment and not needed for their own, individual work.
Over the years, my team and I have converged to an open source business model that works particularly well in the data science market.
Our open source platform provides all the functionality that individual data scientists need. Unlike the classic open-core model, we provide no additional paid functionality for the open source platform — and no additional functionality is needed for real-world use.
Offering a ready-to-use commercial distribution is not an option for us since we provide a simple-to-install distribution of our entire open source product. This is important to the data scientists who are our key users — and who would usually rather be solving data science problems than building applications from scratch.
Our commercial product, however, provides functionality that enables larger data science teams to collaborate and put their workflows built with the open source platform into production. This is all functionality that an individual user of the open source platform does not need and only provides value when the software is used in the context of a production environment.
Obviously, typical enterprise functionality, such as versioning, integrations with existing security setups, etc., is also available on the commercial product.
One particularly pronounced aspect of this setup is the division of the software into two separate packages based on the needs of the users. The open source platform caters to the needs of individual users, and the commercial server software is geared toward the needs of businesses and teams. This makes it extremely easy to separate the two — the requirements as well as the audience are fundamentally different.
This division also protects against a common fear among commercial open source software vendors of cloud providers taking away their distribution business by providing hosted versions of the open source software. Hosting the open source platform does not add much value to the individual. The piece that would be worth hosting is commercial and hence not as easy to host by others.
It also brings the commercial benefit of only requiring a very lightweight marketing effort and short-cutting the early stages of the typical customer journey: the open source analytics platform is usually already heavily used within the organization when our commercial team gets involved.
Unstructured data was the weird thing a couple of years ago and is now just standard. Data science experts often want/need to try out new methods that are only available in rapidly evolving open source environments, but when it turns out that these methods are effective on real-world problems, they are then hard to productionize.
Remember big data? A “must have” a few years ago and now just another piece of the puzzle. Deep learning, once the hype cools down, will just be another tool in the machine learning toolbox.
In an innovation-driven field such as data science, having to wait for a proprietary software vendor to offer its own implementations of new technologies can take way too long. An open source community reacts much faster to new developments — and that can be a big benefit to your product.
In the case of our open source platform, this can take on various forms, such as new modules (“nodes”) created by the community to enable new methods or integrate the platform with new software. We can pick and choose which of these community contributions to officially support, giving users confidence that they can trust official packages, while not restricting access to new innovations.
Connecting the user and customer journeys
As with many other open source business models, the open source ecosystem also provides a different avenue for marketing and the early parts of the customer journey.
Data scientists inside organizations start their individual projects using the open source software without having to go through lengthy business budgeting, approval, and purchasing processes. They often advocate the use of the commercial complement and have already validated the business value from using the open source part, essentially bypassing typical early tool evaluation processes.
Moreover, since open source software is used increasingly for teaching data science in academia, new entrants to the industry bring along the core expertise needed to hit the ground running.
The diagram below summarizes the two journeys and how open source usage within an organization often helps bypass the high-risk, early stages of the customer journey.
Raising awareness about the commercial server is more of a marketing activity but focuses on two different areas: first, ensuring open source users are aware which aspects of their organization can benefit from the Server and secondly, making sure KNIME is known to decision-makers as an enterprise-ready, data science solution.
From a marketing perspective, this means that we have to ensure the open source community is aware of the value the commercial piece provides to the company, but of course, we do not directly sell to them.
Not surprisingly, our numbers clearly indicate that chances of converting commercial interest into a successful customer relationship are far higher when we already have a group of active open source users in-house.
Right now, the majority of our business still comes from inbound requests by happy KNIME Analytics Platform users who are aware of KNIME Server and see the added value of paying for it. So if your company is pondering going into the open source world, I say go for it!
Get the TNW newsletter
Get the most important tech news in your inbox each week.