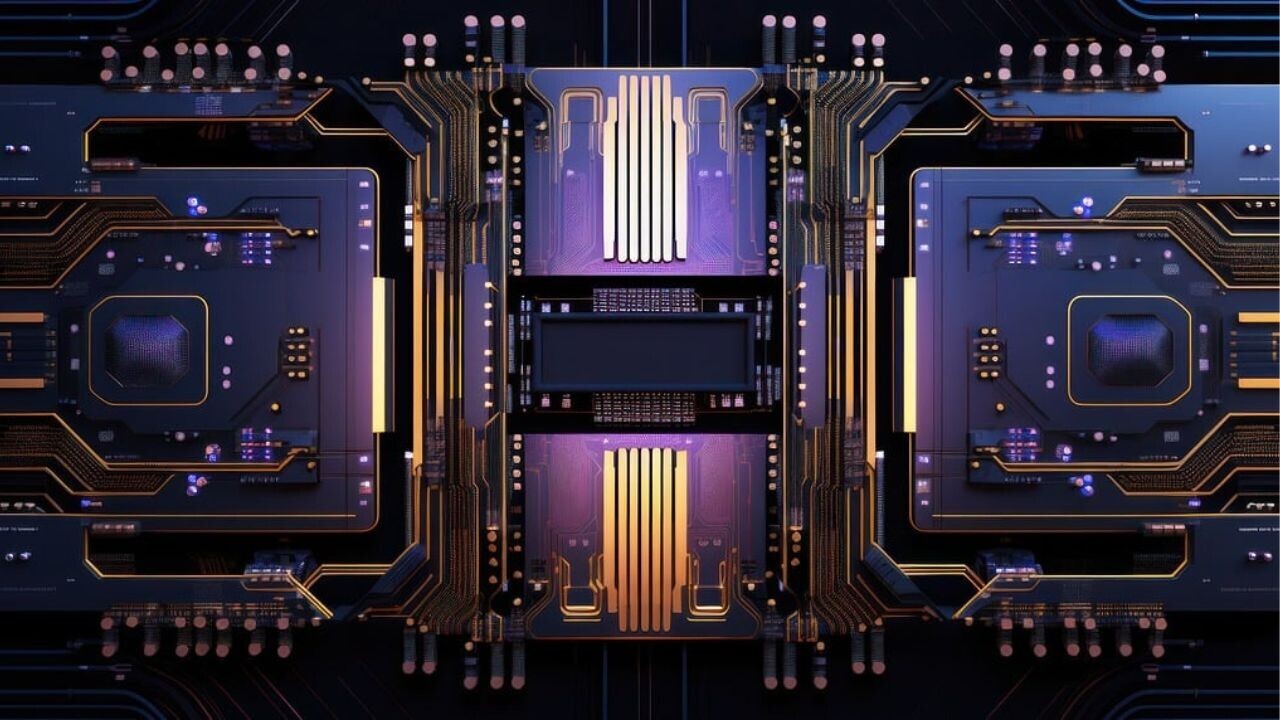
In new research, Google DeepMind has demonstrated that its AI can help accelerate the development of quantum computers — taking one step further in combining two of the most disruptive technologies.
DeepMind worked together with UK-based Quantinuum to solve a key challenge in fault-tolerant quantum computers: reducing the number of T gates.
T gates are essential in implementing a quantum circuit — a network of gates that manipulates qubits to generate algorithms. However, T gates are also the most expensive and most resource-intensive gates of the network.
To address this, the team developed AlphaTensor-Quantum, an extension of DeepMind’s AlphaTensor, the first AI system that can discover efficient algorithms for tasks such as matrix multiplication.
AlphaTensor-Quantum is an AI model that leverages the relationship between optimising T-count and tensor decomposition, using deep reinforcement learning.
In contrast to existing approaches, the model can incorporate domain-specific knowledge about quantum computation as well as use “gadgetisation” techniques, which implement alternative gates by introducing additional qubits and operations. This way, the AI can significantly reduce the number of T gates.
According to the researchers, AlphaTensor-Quantum outperforms existing systems for T-count optimisation and is as efficient as the “best” human-designed solutions across numerous applications. It can also save “hundreds of hours” of research by optimising the process in a fully automated way, the team says in the paper.
“On a representative standard benchmark set of circuits, AlphaTensor-Quantum improves the cost by 37% on average on the existing state of the art obtained by human-crafted heuristics,” Konstantinos Meichanetzidis, head of product development at Quantinuum, told TNW.
DeepMind and Quantinuum envision applications in quantum chemistry and related fields, and suggest that possible future research could focus on improving the algorithm’s neural network architecture.
“In general, the method can be readily applied to any given circuit independent of application, and the improvements over the baselines correspond directly to space and time cost of the quantum algorithm under consideration,” Meichanetzidis said.
Update (11:30AM CET, February 27, 2024): The article has been updated to include the comments from Quantinuum.
One of the themes of this year’s TNW Conference is Ren-AI-ssance: The AI-Powered Rebirth. If you want to go deeper into all things artificial intelligence, or simply experience the event (and say hi to our editorial team), we’ve got something special for our loyal readers. Use the code TNWXMEDIA at checkout to get 30% off your business pass, investor pass or startup packages (Bootstrap & Scaleup).
Get the TNW newsletter
Get the most important tech news in your inbox each week.