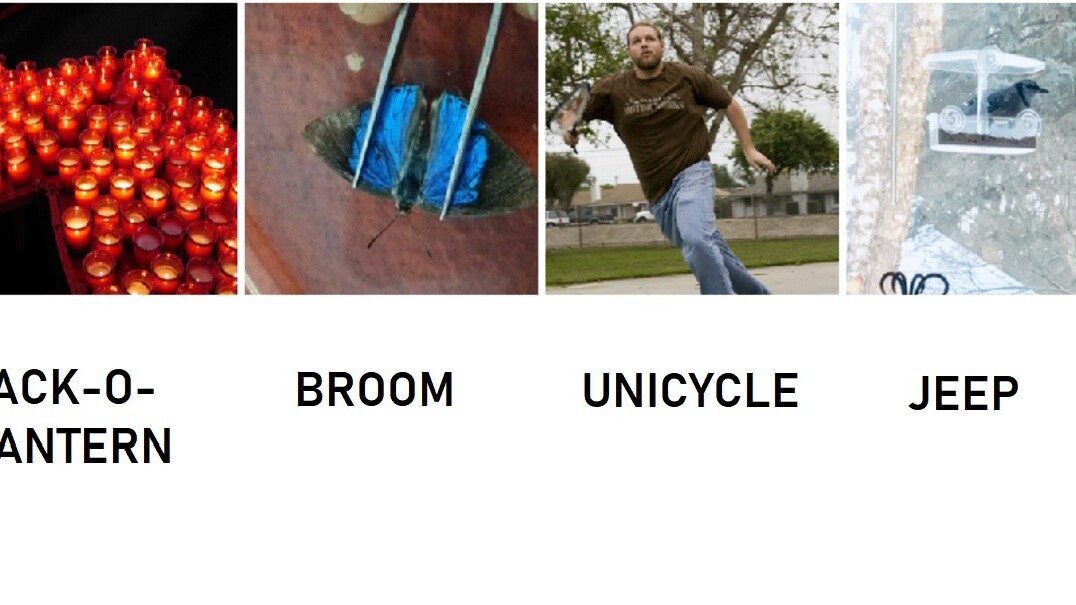
You can’t fool all the people all the time, but a new dataset of untouched nature photos seems to confuse state-of-the-art computer vision models all but two-percent of the time. AI just isn’t very good at understanding what it sees, unlike humans who can use contextual clues.
The new dataset is a small subset of ImageNet, an industry-standard database containing more than 14 million hand-labeled images in over 20,000 categories. The purpose of ImageNet is to teach AI what an object is. If you want to train a model to understand cats, for example, you’d feed it hundreds or thousands of images from the “cats” category. Because the images are labeled, you can compare the AI’s accuracy to the ground-truth and adjust your algorithms to make it better.
ImageNet-A, as the new dataset is called, is full of images of natural objects that fool fully trained AI-models. The 7,500 photographs comprising the dataset were hand-picked, but not manipulated. This is an important distinction because researchers have proven that modified images can fool AI too. Adding noise or other invisible or near-invisible manipulations – called an adversarial attack – can fool most AI. But this dataset is all natural and it confuses models 98-percent of the time.
According to the research team, which was lead by UC Berkeley PhD student Dan Hendrycks, it’s much harder to solve naturally occurring adversarial examples than it is the human-manipulated variety:
Recovering this accuracy is not simple. These examples expose deep flaws in current classifiers including their over-reliance on color, texture, background cues.
ImageNet is the brainchild of former Google AI boss Fei Fei Li. She started work on the project in 2006, and by 2011 the ImageNet competition was born. At first, the best teams achieved about 75-percent accuracy with their models. But by 2017 the event had seemingly peaked as dozens of teams were able to achieve higher than 95 percent accuracy.
This may sound like a great achievement for the field, but the past few years have shown us that what AI doesn’t know can kill us. This happened when Tesla’s ill-named “Autopilot” confused the white trailer of an 18-wheeler for a cloud and crashed into it resulting in the death of its driver.
So how do we stop AI from confusing trucks with clouds, or turtles for rifles, or members of Congress for criminals? That’s a tough nut to crack. Basically, we need to teach AI to understand context. We could keep making datasets of adversarial images to train AI on, but that’s probably not going to work, at least not by itself. As Hendrycks told MIT’s Technology Review:
If people were to just train on this data set, that’s just memorizing these examples. That would be solving the data set but not the task of being robust to new examples.
The ImageNet-A database finally exposes the problem and provides a dataset for researchers to work with, but doesn’t solve it. The ultimate solution, ironically, may involve teaching computers how to be more accurate by being less certain. Instead of choosing between “cat” or “not cat,” we need to come up with a way for computers to explain why they’re uncertain. Until that happens — which may take a completely new approach to black box image-recognition systems — we’re likely still a long way from safe autonomous vehicles and other AI-powered tech that relies on vision for safety.
Get the TNW newsletter
Get the most important tech news in your inbox each week.